Best Practice Presentation: Lessons learned on data discovery, integration and ingestion in AGRIS.
Title: | Lessons learned on data discovery, integration and ingestion in AGRIS. |
Date: | 2020-09-22 15:00 |
Slides: |
slides.pdf |
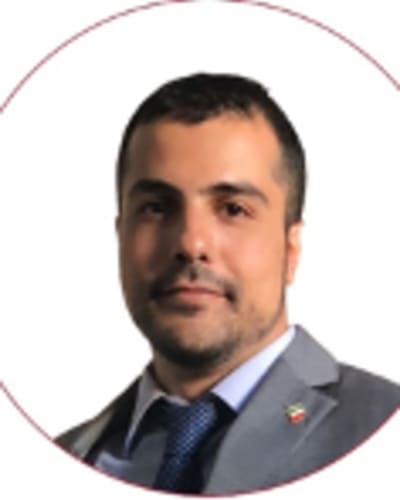
Fabrizio Celli
Food and Agriculture Organization of the United Nations
Fabrizio Celli received the MSc in Computer Science and Engineering from the University of Rome "Roma Tre" in 2009. He also received the BSc in Psychology from the University of Rome "La Sapienza" in 2015. Currently working for the Food and Agriculture Organization of the United Nations (FAO), he has 11 years of experience as a software engineer. His major skills are web application development, search engines, linked open data, big data, machine learning, and IT security. He has interests in Neuroscience and Theoretical Physics.
Food and Agriculture Organization of the United Nations
Fabrizio Celli received the MSc in Computer Science and Engineering from the University of Rome "Roma Tre" in 2009. He also received the BSc in Psychology from the University of Rome "La Sapienza" in 2015. Currently working for the Food and Agriculture Organization of the United Nations (FAO), he has 11 years of experience as a software engineer. His major skills are web application development, search engines, linked open data, big data, machine learning, and IT security. He has interests in Neuroscience and Theoretical Physics.
Abstract
AGRIS, the International System for Agricultural Science and Technology, is a multilingual bibliographic database that connects users directly to a rich collection of research and worldwide technical information on food and agriculture. AGRIS has a network of almost 500 data providers that have contributed to the growth of the database since 1974. Considering the high number of data providers and their diversity, AGRIS must cope with several challenges to integrate and ingest data from all existing data sources, like the existence of several metadata formats and different standards in terms of metadata quality. In addition, with the continuous growth of open repositories and the publication of APIs to harvest data, AGRIS has started the process of automating the ingestion of data in its database. The adoption of machine learning algorithms (neural networks) and controlled vocabularies like AGROVOC play a key role in content understanding and classification of high-volume data, allowing correct data discovery and integration.
Intended audience: librarians, information managers, anyone who wants to contribute to AGRIS